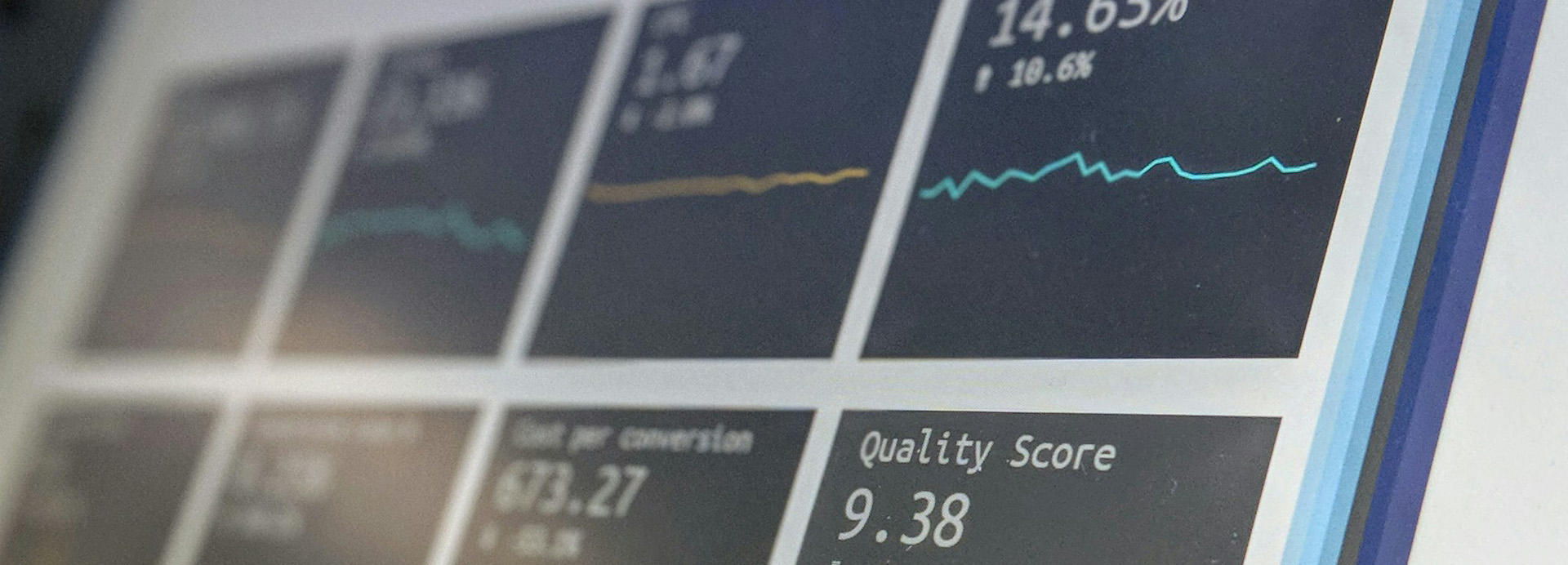
AI-Driven Gap Analysis in Winning a Grant
7/6/2024 • Scott LoveBlue Raven conducted a comprehensive Gap Analysis for a leading non-profit organization dedicated to mental health. The grant, funded by a private donor and measured in tens of millions of dollars, specifically called for a technology plan focused on improving operational performance of its awarded non-profit recipients.
Our client called on us to evaluate their current use of technology and lay out a roadmap for innovation in support of securing the grant.
The breadth of the organization proved to be our greatest challenge: in addition to a national, central team of roughly 100 employees, the organization had local and state affiliates across the country - each of which used technology differently and had varying needs. We needed to assess dozens of teams and take input from potentially hundreds of individuals.
To bring the level of effort within reach, Blue Raven used AI and Large Language Model tools - namely ChatGPT at the time - to synthesize interview results and, as an added value, created a platform for our client that allowed for efficient knowledge-sharing and future investigations.
Interviews as Source for ChatGPT
Blue Raven conducted more than 60 group interviews with stakeholders across various levels, including senior management, mid-level employees, and operational staff.
Intending to use AI to analyze results, we knew we needed good source data:
- We used the same script of questions in each interview.
- We identified each speaker throughout interviews and deliberately used their names when presenting and addressing questions.
- We used “reflective listening” to both confirm understanding and to provide the LLM with summaries throughout.
- In the beginning of each interview, we clearly called out preferred terminology for a given group. (In some cases, some teams referred to systems and platforms differently than other teams.)
All of this helped ensure that our toolset could follow the flow of a conversation and compare against other interviews. We recorded each interview and transcribed results into a structured text-based data source.
Analysis Methodology
We broke our analysis into three categories:
- User-Facing Elements: those aspects that impacted end users of a given process or system.
- Technology-Facing Elements: issues that our client’s IT organization had to manage (for example, system backups or authentication).
- Business-Stakeholder Elements: specific requirements our client’s leadership team had, particularly those focused on reporting.
Our analysis, based on interviews and some additional direct systems review, synthesized the following data points (when applicable and supported within the research) for each system within our client’s technology portfolio:
- Overall system usage by team, as reported by those teams
- System “business importance” - how mission-critical a given system was
- General sense of “business friction” - reported in subjective terms, of how well a given system supported its functions within a team
- Technical debt facing ongoing support of a given system
- Security risks for a given system
- Operational overhead for maintaining a system
- Confidence in data - its structure and how “clean” the data pool was
- Future potential for operational improvement - how rigid or flexible a system was and how well it might support future evolution, innovation, etc.
We then took an additional step and categorized each system across identified process workflows: some systems supported just one business function whereas others might support a dozen or more. (For example, some teams would send invoices and use a specific accounting platform to do so.)
Results - and Added Value
Our findings revealed various opportunities for significant improvements in our client’s technology infrastructure and provided a clear case for which systems should be improved, which should be consolidated or eliminated, and which were of less critical priority.
The work yielded a further benefit as well: the interview engine can be queried to address specific additional questions and used again in another round of interviews to compare against initial baseline results.
As part of our grant application, we demonstrated having put into place not only measured results, key performance indicators, and recommendations but also a means of driving IT agendas via subsequent polling rounds and data collection.